Curse of dimensionality
The number of instances increases exponentially to achieve the same explanation ability when the number of variables increase.
변수의 개수가 선형적으로 늘어날 때, 동일한 설명력을 갖기 위해서 필요한 객체 수는 지수적으로 늘어난다.
ex) 두 점 사이 거리가 1인 정보를 표현하기 위해, 1차원에서는 점 2개, 2차원에서는 점 4개, 3차원에서는 점 8개 필요
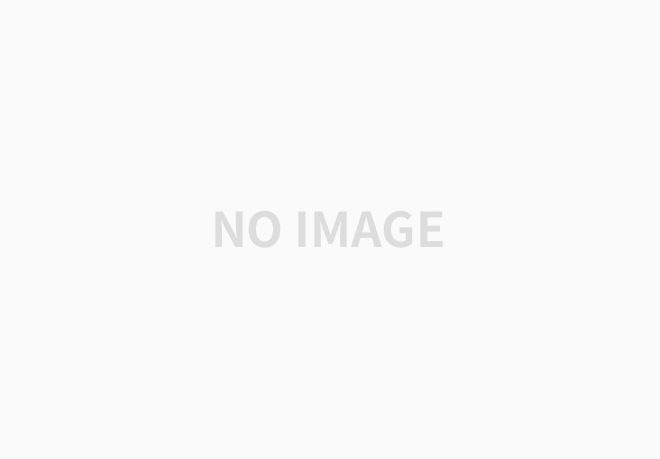
Sometimes, an intrinsic dimension is relatively low compared to original dimension.
객체의 본질적인 정보를 보존하는 내재적인 차원의 수는 실제 차원의 수보다 적다.
ex) 256차원(16*16) 데이터를 2차원으로 축소해도 설명력을 가짐
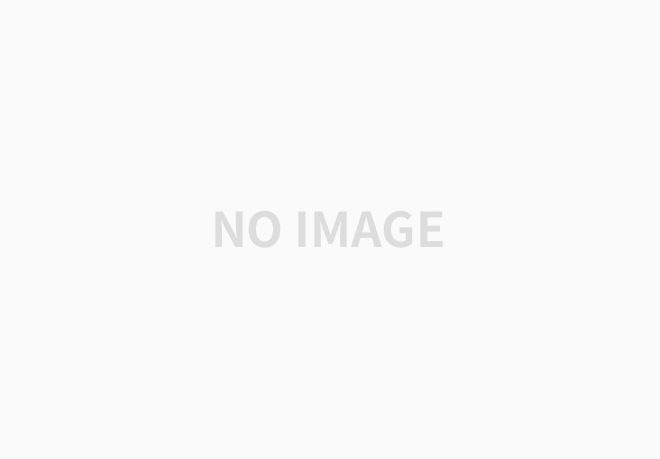
Problems caused by high-dimensionality
- Increase the probability of having noise in data → degenerate the prediction performance
- Increase computational burden for training/applying prediction models
- Require more number of examples to secure generalization ability of prediction model
To resolve the curse of dimensionality
- Utilize domain knowledge
- Use a reqularization term in objective function(ex. L1, L2)
- Employ a quantitative reduction technique
Dimensionality Reduction
Purpose
- Identify a subset of variables that best fit the model
Effect
- Remove correlations between variables
- Simplified post-processing
- Remove redundant or unnecessary variables while keeping relevant information
- Visualization can be possible
Techniques
- selection vs extraction
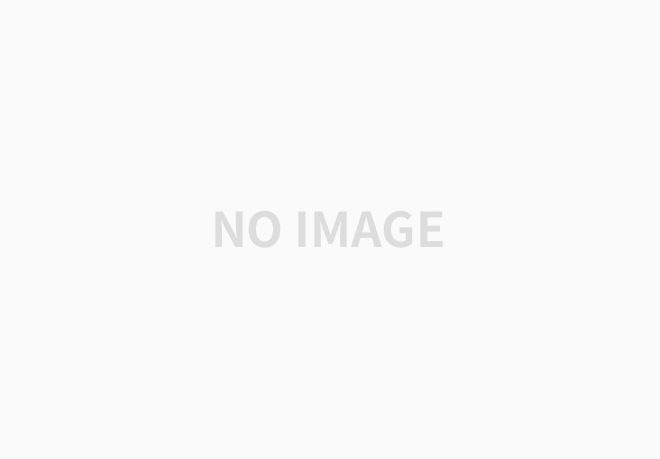
출처
- 01-1: Dimensionality Reduction Overview
https://youtu.be/ytRmxBvyGG0
'Business Analytics' 카테고리의 다른 글
AARRR 개념 (5) | 2024.01.10 |
---|---|
1-3. 차원축소 - Feature Extraction(1); PCA, MDA (0) | 2023.01.11 |
1-2. 차원축소 - Feature Selection (0) | 2022.06.26 |
댓글